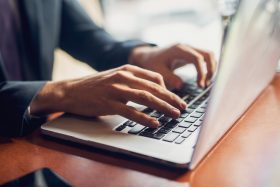
Enhanced MLI syllabus
- The MLI is now comprised of 2 levels
- Self-paced Primers & Introduction Suite on Registration
- 8 modules over 36 lectures
- Dedicated Faculty Support is available every step of the way, for the Primers and weekly lectures via the student forum.
- Students who require extra help can schedule calls directly with the MLI faculty.
- End of Module Online Tests (Students get two attempts at each module test)
- Practical final project and a final exam which can be taken from any global location online using our live invigilation platform.
Self-paced Primers & Introduction Suite
On registration students have access to the MLI Primers & Introduction Suite. This is a self-paced portal on the mathematics for machine learning and python techniques,
Dedicated Faculty Support is available every step of the way, for the Primers and weekly lectures via the student forum. Students who require extra help can schedule calls directly with the MLI faculty.
Our team will monitor and respond to any queries promptly.
Self-paced: Mathematics for Machine Learning
Students who require extra help with the Primers can schedule calls directly with the MLI faculty. Our team will monitor and respond to any queries promptly.
Overview
- Calculus
- Linear Algebra
- Analytic Geometry
- Matrix Decompositions
- Vector Calculus
- Probability
- Probability Distributions
- Continuous Optimization
- Risk and Pricing
Self-paced: Python for Data Science and Artificial Intelligence
Students who require extra help with the Primers can schedule calls directly with the MLI faculty. Our team will monitor and respond to any queries promptly.
Overview
Python is the de factolingua franca of data science, machine learning, and artificial intelligence. Familiarity with Python is a must for modern data scientists.
The MLI Python Primers are designed to take you from the very foundations to state-of-the-art use of modern Python libraries.
You will learn the fundamentals of the Python programming language, play with Jupyter notebooks, proceed to advanced Python language features, learn to use distributed task queues (Celery), learn to work with data using NumPy, SciPy, Matplotlib, and Pandas, examine state-of-the-art machine learning libraries (Scikit-Learn, Keras, TensorFlow, and Theano), and complete a realistic, real-life data science lab.
Syllabus:
- The fundamentals of the Python programming language and Jupyter notebooks
- Jupyter notebooks
- The Python syntax
- Data types, duck typing
- Data structures: lists, sets, and dictionaries
- Data types
- Advanced Python features; distributed tasks queues with Celery
- List comprehensions
- Lambdas
- Objects
- The Global Interpreter Lock (GIL)
- Multithreading and multiprocessing
- Distributed task queues with Celery
- Python libraries for working with data: NumPy, SciPy, Matplotlib, and Pandas
- Multidimensional arrays in NumPy
- Linear algebra and optimisation with SciPy
- Data visualisation in Matplotlib
- Time series data
- Dealing with Pandas DataFrames
- Machine Learning with Scikit-Learn; Deep Learning with Keras, TensorFlow, and Theano
- Overview of machine learning
- Introduction to Scikit-Learn
- Keras and TensorFlow
- Introduction to Theano
Self-paced: Advanced Python Techniques
Students who require extra help with the Primers can schedule calls directly with the MLI faculty. Our team will monitor and respond to any queries promptly.
Advanced Python Features and Putting them to use in Practice
- Algorithmics and graph theory
- Prime numbers
- Cryptography
- Blockchain
- Distributed Computing with Python
High Performance Python
Outline syllabus
· Profiling
· The use of NumPy and SciPy over pure python
· The importance of optimised NumPy and SciPy
· The use of Cython and ctypes to integrate compiled code
· Just In Time Compilation using Numba
· Distributed computing frameworks
· Numerical precision and speed
· Using specialised instead of generalised algorithms
· NAG Library for Python
Abstract
Python is a superb prototyping language that allows us to develop high quality data analyses and simulations in a relatively short amount of time. The cost we pay for this is performance. Python is essentially an interpreted and single threaded language which puts severe limitations on its speed. In this session we will learn a range of techniques that will allow us to discover which parts of our Python code are slow and what we can do to speed things up.
Level 1: Machine Learning Institute Certificate in Finance (MLI)
Dates:
- Level 1 Starts: Tuesday 19th November 2024
- Lectures Start at 18.00 UK Time
Module Tests:
Students are required to take an on-line multiple choice test at the end of each module, counting towards the final grade. After completion of Module 8 students sit the unseen written examination, held in the examination suite for London-based students. All other students sit the exam virtually at their own desktop. The exam is taken on the same day worldwide.
Module 1 – Supervised Learning:
In this module, the concepts related to algorithmically learning from data are introduced. The candidates are given an early taste of a supervised machine learning application before going through the fundamental building blocks starting from linear regression and classification models to kernels and the theory underpinning support vector machines and then to the powerful techniques of ensemble learning.
Module 1 deals with the fundamentals of machine learning. Practical exercises, examples and projects will follow in later modules. Module 1 will include a mock test, to assist students with the end of module online tests.
- Lecture 1: Statistical estimation theory and introduction to machine learning
- Lecture 2: Linear regression
- Lecture 3: Regularized linear regression and ensemble methods
- Lecture 4: Introduction to Bayesian modelling
End of Module 1 Online Test
Module 2 – Deep Learning:
Deep Learning has been the driving engine behind many of the recent impressive improvements in the state of the art performance in large scale industrial machine learning applications.
This module can be viewed as a natural follow-up from the previous module on Neural Networks. First, the background and motivations for transitioning from traditional networks to deeper architectures are explored. Then the module covers the deep feedforward architecture, regularisation for deep nets, advanced optimisation strategies and the CNN Architecture.
The assignments of this module will be highly practical with ample opportunity to experiment on financial and non-financial data sets and become familiar with the latest open-source deep learning frameworks and tools.
- Lecture 5: Machine Learning and the Perceptron
- Lecture 6: Backpropagation, Optimisation and Regularisation
- Lecture 7: Neural Networks in Banking and Finance
- Lecture 8: Predict Corporate Bond Spread Changes
- Lecture 9: Deep learning volatility
End of Module 2 Online Test
Module 3 – Unsupervised Learning and Alternative Data
- Lecture 10: Introduction and dimensionality reduction
- Lecture 11: Clustering algorithms
- Lecture 12: PCA and autoencoders
- Lecture 13: Alternative data
End of Module 3 Online Test
Module 4 – Practitioner’s Approach to Machine Learning:
This module focuses on the practical challenges faced when deploying machine learning models within a real life context.
Each session in this module covers a specific practical problem and provides the candidates with guidance and insight about the way to approach the various steps within the model development cycle, from data collection and examination to model testing and validation and results interpretation and communication.
- Lecture 14: Hardware of machine learning
- Lecture 15: Reproducibility and Deployment of Data Science Workflows
- Lecture 16: Feature engineering and model tuning
- Lecture 17: Differential machine learning
End of Module 4 Online Test.
End of MLI Level 1
Level 1 Faculty Q&A Sessions: 18.00 GMT & 18.00 EST
Module Tests:
Students are required to take an on-line multiple choice test at the end of each module, counting towards the final grade. Students get two attempts at each module test.
The second module test attempt can be taken at any point before the final examination, module tests do not need to be taken in any particular order.
After completion of Module 8 students sit the unseen written examination virtually at their own desktop. The exam is taken on the same day worldwide. The MLI is a career-enhancing professional qualification, that can be taken worldwide.
Level 2: Machine Learning Institute Certificate in Finance (MLI)
Dates:
- Level 2 Starts: Tuesday 18th February 2025
- Lectures Start at 18.00 UK Time
Module 5 – Reinforcement Learning
- Lecture 18: Reinforcement Learning: introduction
- Lecture 19: Reinforcement Learning: implementation
- Lecture 20: Reinforcement learning for market making and wealth management
- Lecture 21: Reinforcement learning for optimal order execution
End of Module 5 Online Test
Module 6 – Time Series:
Time series data is an invaluable source of information used for future strategy and planning operations everywhere from finance to education and healthcare. You will be walked through the core steps of building, training, and deploying your time series forecasting models. You’ll build a theoretical foundation as you cover the essential aspects of time series representations, modeling, and forecasting before diving into the classical methods for forecasting time series data.
- Lecture 22: Financial time series data
- Lecture 23: Time series analysis
- Lecture 24: Practical lab session
- Lecture 25: Introduction to time series signatures
End of Module 6 Quizzes.
Module 7 – NLP, Generative AI & Large Language Models
- Lecture 26: Training LLMs for Quant Finance
- Lecture 27: GPT-3: Key Concepts & Use Cases
- Lecture 28: Deep learning for text
- Lecture 29: Foundation NLP Models for ESG data extraction
- Lecture 30: Attention, transformers, and BERT
- Lecture 31: Generative modelling, variational autoencoders, and GANs
- Lecture 32: A data-driven market simulator for small data environments
End of Module 7 Online Test
Module 8 – Quantum Machine Learning
- Lecture 33: Introduction to quantum computing
- Lecture 34: Variational circuits as machine learning methods
- Lecture 35: Quantum models as kernel methods
- Lecture 36: Potential quantum advantages
End of Module 8 Online Test
Level 2 Faculty Q&A Sessions: 18.00 GMT & 18.00 EST
Lifelong Learning: Half day workshop: Large Language Models in Financial Markets
Module Tests:
Students are required to take an on-line multiple choice test at the end of each module, counting towards the final grade. Students get two attempts at each module test.
The second module test attempt can be taken at any point before the final examination, module tests do not need to be taken in any particular order.
After completion of Module 8 students sit the unseen written examination virtually at their own desktop. The exam is taken on the same day worldwide. The MLI is a career-enhancing professional qualification, that can be taken worldwide.
Examination & Final Project
Final Examination:
Examination Preparation Session: Tuesday 27th May 2025
Examination Date: Tuesday 10th June 2025
- Candidates will sit a formal examination on a computer. The exam is taken online by students globally.
Final Project:
Hand in Date: Friday 27th June 2025
At the end of the programme, candidates apply the theoretical and practical skills acquired to a real world application within the financial services industry.
The assessment will take into account the quality and the originality of the work as well as the clarity of its presentation.