In-house
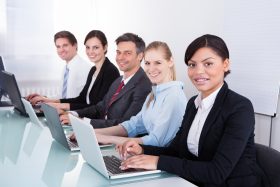
In-house & Bespoke Training
We draw on the experience of an extensive network of industry and academic experts to deliver courses tailor-made for you.
If you need an entirely new training package designed to your specific needs or a course delivered at a venue of your choice, our expert trainers will come to your organisation and provide bespoke training on topics such as:
- The world of LIBOR transition
- Machine Learning & AI
- Blockchain
- Stress Testing
- Interest Rate Modelling
- CVA, KVA & XVA
- Initial Margin Requirements
- Distributed Ledger Technology
- FRTB Fundamental Review of the Trading Book
- MiFID II
- IFRS 9
- Bank Treasury Risk Management & ALM
To enquire about our In-house training services, please contact:
Email : neil@wbstraining.com
Tel: Neil Clive Fowler, +44 (0) 1273 201352
The world of LIBOR transition
The capital markets industry started to prepare for an alternative rate to LIBOR more than three years ago, and now the buy-side must ready itself for several critical milestones ahead. LIBOR, the most widely used interest rate benchmark in the world, is expected to phase out by the end of 2021. More than $350 trillion in notional of cash and derivative securities currently track LIBOR globally, approximately $200 trillion of which is linked to USD LIBOR. The industry started preparations for the transition to an alternative rate more than three years ago and a lot of progress has been made. But the next two years involve several critical milestones and it’s time for buy-side firms to prepare for the transition. In his remarks at the 2019 U.S. Treasury Market Conference, John Williams, President and Chief Executive Officer of the FRBNY, delivered a wakeup call when he said, “The clock is ticking, LIBOR’s days are numbered, and we all need to play our part in preparing the industry for January 1, 2022.”
- Updates on Global Banking Regulatory Oversight
- Reviewing the Sterling LIBOR market
- Reviewing USD LIBOR market
- Across the pond, but so far away: regulators differing approaches
- IRS : adverse tax consequences
- Securities and Exchange Commission on LIBOR transition
- Comparing approaches by SEC, the CFTC and FINRA
- Impact on Products & Reviewing new offerings
- Illustrative example: 1st transaction switching an existing securitization to SONIA
- Key considerations for floating rate notes, RMBS and ABS
- FHFA Supervisory letter interpretation
- ARRC-recommended LIBOR fallback language
- The Devil is in the details: Documentation
- Robust fallback language by market conventions
- Amendments to transactions with less than 100% holder approval
- Amendments to USD LIBOR transactions
- Case Study: FDIC & new transactions
- Key Libor Edge cases
- ZOMBIE LIBOR: What is the regulatory tests of representativeness
- Liquidity: LIBOR-related contracts
- Hedging outstanding LIBOR obligations
- Conduct risks: LIBOR transition
- Case Study: Industry preparedness: “drivers and passengers”
- Accounting recognition of LIBOR transition
- Examining pre-cessation triggers for LIBOR fallbacks
- ARR Calculation methodology and considerations
- ARR spread and term adjustments
- Compounded setting in arrears rate
- Historical mean/median approach
- Market Infrastructure shifts
- What is CME & LCH doing? Just leading the way, that’s all.
- Changes to clearing, collateral, and technology
- Impact of LIBOR transition on futures market contributors
- Complications for buy-side firms: A focus on risk management
- Portfolio turnover rates
- New LIBOR-linked risks
- “Tough legacy transactions”
- Deep-dive: SOFR & Emerging Competitors
- The publication of SOFR averages, SOFR Index examination, SOFR Term Structure
- SOFR volatility spikes & The repo market
- A review of the Federal Reserve’s abilities for interventions to stabilize SOFR
- Looking at AMERIBOR of the American Financial Exchange
- ICE Bank Yield Index of ICE Benchmark Administration
- Transitioning to the new ARR
- Practical Implementation Checklist for SOFR Adoption,
- SOFR + a credit risk premium
- Specific product considerations
- Forward rate notes:
- LIBOR fallback language for new residential adjustable-rate mortgages
- CLOs new LIBOR fallback language & basis risk
- Student-loan asset-backed securities (SLABS)
- The quality (or absence) of LIBOR fallback language impact on pricing and valuation
- Residential ARM participants
- Acumen & leadership: The market has spoken; analysis
- Leading calculation agent & data provider: Bloomberg’s
- Bank’s & Buy side’s “holdout” behavior
- Action plan: What should market participants be doing right now?
- Steps for existing & newly acquired LIBOR-based positions
- Dealing with difficult products: “Tough legacy” transactions are a significant concern for financial stability
- Transactions that lack robust/ or any LIBOR fallback language
- Practical issues such as unanimous consent requirements
- Political considerations for retail market participants
- Citibank’s perpetual preferred securities: A true headache for market participants
- ARRC: Enacting jurisdictional law in New York and the federal level.
- Deep-dive operations for newly acquired LIBOR-based positions:
- Implement risk-management procedures
- LIBOR cessation and whether they can be amended (with or without a holder’s consent).
- Setting limits on the acquisition of new LIBOR-based positions.
- For existing LIBOR-based positions:
- Inventory management for LIBOR-based positions
- Performing risk management to analyze historic portfolio
- Implementing a risk-based approach for LIBOR transition
- They Live: For those LIBOR-based positions on the portfolio at the end of 2021:
- Documentation: provisions regarding LIBOR use, LIBOR fallbacks, amendments, etc.
- Developing a plan to: Initiate, respond to, and track on efforts to amend LIBOR-use provisions and/or old fallbacks
- Operationalize LIBOR fallbacks so they are administered correctly
- For those LIBOR-based positions that may be affected in terms of valuation, pricing, modeling or other characteristics, develop plans to, among other things:
- Update valuation and pricing methodologies and procedures, and
- Update models to incorporate LIBOR alternatives (including for discounting, etc.).
- For operations and compliance: Identify systems that need to be updated to use non-LIBOR rates such as for discounting, interest computations, modeling and treasury management and develop a plan for updating them
- Develop a plan to respond to regulators’ inquiries about LIBOR transition planning
- Risk and other disclosures – to explain risks related to LIBOR transition
- Valuation procedures – to account for possible valuation impacts of weak or nonexistent LIBOR fallback provisions
- Policies and procedures for approving new LIBOR-based positions
Machine Learning in Finance
What delegates will require:
Laptops required for all or in groups (we encourage collaboration during the tutorials.) Solutions to problems, including programming assignments, will be provided during the training, so you can follow them. Python is a (de facto) lingua franca of data science and machine learning, so we’ll use it as our primary programming language. We advise that you install the Anaconda Python distribution (64-bit, version 5.0.0, the Python 3.6 variant at the time of writing) by Continuum Analytics. This distribution includes the following libraries, some of which we may use during the training:
- NumPy — the fundamental package for scientific computing with Python; it contains, among other things, a powerful n-dimensional array object;
- SciPy (pronounced “Sigh Pie”)—Python-based ecosystem of open-source software for mathematics, science, and engineering;
- SciKits — SciPy Toolkits;
- Pandas — Python Data Analysis Library;
- StatsModels— Statistics in Python;
- Keras — the Python deep learning library, a high-level neural networks API running on top of TensorFlow, CNTK, or Theano.
Prerequisites:
There are no formal prerequisites for the course, and we’ll endeavour to explain the foundational concepts during the training if required. However, since data science and machine learning rely on the following disciplines, it is good to brush up on:
- Linear algebra — we are dealing with datasets consisting of many data points and algorithms with many (hyper)parameters; linear algebra is the essential language in this multivariate setting;
- Probability theory — many of the models are versed in the language of probability: for example, disturbances in linear regression models are random variables; frequentist likelihoods and Bayesian priors and posteriors are probabilities; Somewhat less pertinently:
- Information theory — this branch of applied mathematics is concerned with quantifying how much information is present in our inputs; in machine learning we are concerned with extracting as much information as possible;
- Numerical computation — many of the algorithms rely on numerical methods rather than analytical solutions; in practice care is needed to avoid numerical issues such as overflow and underflow, poor conditioning, etc.;
- Optimisation theory — much of machine learning is concerned with optimising (hyper)parameters and therefore utilises the machinery from optimisation theory, such as gradient-based optimisation.
In order to practise machine learning, one needs
- A working knowledge of a convenient programming language, such as Python or R;
- Familiarity with relevant libraries.
- During our trainings, all code demonstrations will be using Python.
Training topics:
- Lecture 1: interpretations of probability— classical, frequentist, Bayesian, axiomatic
- Lecture 2: statistical inference and estimation theory
- Tutorial 1: statistical inference and estimation theory
- Lecture 3: introduction to linear regression: a geometric perspective
- Lecture 4: interpreting the linear regression, multicollinearity
- Tutorial 2: linear regression
- Lecture 5: from statistics to machine learning: bias-variance trade-off, under- and overfitting
- Lecture 6: cross-validation and shrinkage methods
- Tutorial 3: demo of cross-validation and shrinkage methods
- Lecture 7: optimisation, gradient descent
- Lecture 8: neural networks and deep learning
- Tutorial 4: neural networks
Sound Stress Testing and Scenario Analysis
Outline:
Stress testing has become an important analysis practiced across multiple industries as part of a robust enterprise-wide risk management framework. Since the global financial crisis there has been a greater focus on the regulatory aspects of the implementation and rigorous stress testing program across all major financial institutions. Financial institutions have responded to the crisis and this intensified regulatory scrutiny over the last several years, dramatically increasing their commitment to internal stress testing programs. Throughout this course participants will develop methods to build well structured stress tests and scenario analysis. Exploring and building detailed case studies and realistic stress tests.
Topics:
1) Methods to build well-structured stress tests and scenario analysis: which technique should we use (Bayesian nets and other methods)?
2) The three main applications:
a. single – transaction analysis
b. asset allocation
c. large and complex portfolios
3) How to root stress testing in solid financial theory
4) How to handle the dimensionality curse (ie, how to cascade a stress view from a small number of risk factors to many asset prices)
5) How to handle the consistency requirement between stress and no-stress risk measures
6) How to associate an approximate probability to the scenario
7) How to carry out sensitivity analysis
8) How to carry out a Monte Carlo simulation for stress analysis
9) Bells and whistles – ie, how to refine the results and extract additional information
Case Studies and Realistic Stress Tests:
During the course the delegates will build a realistic stress-testing scenario chosen by the delegates (Hard Brexit? Italian banking crisis? North Korea crisis?) both for a complex portfolio and for a specific transaction (eg, Hard Brexit and the UK yield-curve steepener trade).
During the construction of the scenario, the insights and techniques learnt during the course will be used in practice. The delegates will go back to their offices knowing how to carry out a realistic scenario themselves.
Modules
Module 1
Modern Market structure looking beyond 2020: The rise of alternative technology, marketplaces, and products such as exchange traded derivatives, and crypto currencies.
- Exchanges, Clearing houses, and Collateral
- Exchange traded & OTC derivative landscape
- Crypto currencies dynamics, offerings, and eco system
- Blockchain, AI, and machine learning in trading, finance, and operations
Module 2
Regulations, Donald Trump, Brexit, and Red Flag Risk Factors- Studying the impacts on the capital markets
- MiFID II: One of the most seismic regulatory shifts in history affecting everything from research to dark pools
- Who will be Bitcoin’s ultimate regulator: Future state of Crypto currency trading regulation
- The Global LEI Initiative – The LEI is part of a much bigger market structure solution
- Deregulation: a lose-lose game that would have serious consequences for the stability of the world financial system
Module 3
Collateral Tidal Wave: Collateral usage today, tomorrow, and impeding shortfall.
- Account segregation history: MF Global, LSOC, CFTC, CME, and John Corzine
- Instant Payment? Ripple & MoneyGram’s XRP digital currency
- What can China do to help the global collateral exchange?
- Exploring the impact and rule set for non-cleared margin regulation
Module 4
Crypto-currency evolution, use cases, exploring hype vs. sustainability
- Crypto-currency trading, and the regulatory response
- Case Study: Omega One ,leading crypto-asset agency brokerage for asset managers and institutional investors
- Ethereum and SMART contracts: The winning model for settlement in the future.
- Case Study: Silk Road & Dread Pirate- the complete story- and how the illegal marketplace changed market and trading history
Machine Learning in Finance: A Practical View
Outline:
- Using machine learning in the new financial markets big data landscape
- Big Data in Finance Landscape
- Infrastructure and technologyData sources
- Modern data analysis – Structured and Unstructured Data & New Models
- Classical and advanced models
- Machine Learning models in practice
- Machine learning robust modeling
- The future of machine learning in finance
Big Data in Finance Landscape
- Big data in finance landscape: Financial modeling, data governance, integration, NoSQL, batch and real-time computing and storage
- Infrastructure and technology
- New data sources
- Modern data analysis: Structured / Unstructured data and new models
Machine Learning Models
- Supervised learning
- Unsupervised learning
- Reinforcement learning
- Deep learning
- Advanced machine learning models
Machine learning in finance – Practice
- Momentum and Mean Reversion
- Sentiment Analysis
- Asymmetric Trading Strategies
- Non Linear Multi-Factor Models
- High Frequency Trading
- Advanced Machine Learning
Machine learning in finance – Opportunities and challenges
- Algo-Grading 101
- Interpretation
- Data mining biases: overfitting, survivorship and data-snooping
- Robust trading strategies
- The future of machine learning in finance