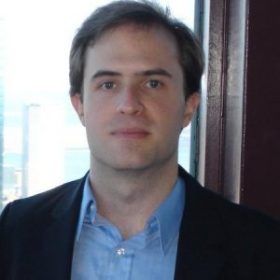
Gordon Ritter:
Founder, CIO, Ritter Alpha, LP
Gordon Ritter: Founder, CIO, Ritter Alpha, LP
Gordon Ritter completed his PhD in mathematical physics at Harvard University in 2007, where his published work ranged across the fields of quantum computation, quantum field theory, differential geometry and abstract algebra.
Prior to Harvard he earned his Bachelor’s degree with honours in Mathematics from the University of Chicago. Gordon is currently a senior portfolio manager at GSA Capital, and leader of a team trading a range of high-Sharpe absolute return strategies across geographies and asset classes. GSA Capital has won the Equity Market Neutral & Quantitative Strategies category at the Eurohedge awards four times, with numerous other awards including in the long-term performance category.
Prior to joining GSA, Gordon was a Vice President of Highbridge Capital and a core member of the firm’s statistical arbitrage group, which although less than 20 people, was one of the most successful quantitative trading groups in history, responsible for billions in pro_t and trillions of dollars of trades across equities, futures and options.
Concurrently with his positions in industry, Gordon teaches courses ranging from portfolio management to econometrics, continuous-time finance, and market microstructure in the Department of Statistics at Rutgers University, and also in the MFE programs at Baruch College (CUNY) and New York University (both ranked in the top 5 MFE programs).
He has published several articles on modern portfolio theory in top practitioner journals including Risk, and academic journals including European Journal of Operational Research.
Abstract:
We address the problem of how to optimally hedge an options book in a practical setting, where trading decisions are discrete and trading costs can be nonlinear and difficult to model. Based on reinforcement learning, a well-established machine learning technique, our model is shown to be flexible, accurate and very promising for real-world applications.
This is joint work with Gordon Ritter.
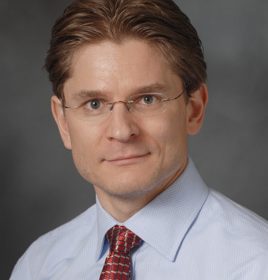
Petter Kolm:
Clinical Full Professor and Director, Courant Institute of Mathematical Sciences, NYU
Petter Kolm: Clinical Full Professor and Director of the M.S. in Mathematics in Finance Program, Courant Institute of Mathematical Sciences, New York University & Partner, CorePoint-Partners.com
Petter Kolm is Clinical Full Professor and Director of the M.S. in Mathematics in Finance Program at the Courant Institute of Mathematical Sciences, New York University, since 2007. He is also Partner at CorePoint-Partners.com. Previously, Petter worked in the Quantitative Strategies group at Goldman Sachs Asset Management, developing proprietary investment strategies, portfolio and risk analytics in equities, fixed income and commodities.
Petter is the co-author of numerous academic journal articles and several well-known finance books including, Financial Modeling of the Equity Market: From CAPM to Cointegration (Wiley, 2006); Trends in Quantitative Finance (CFA Research Institute, 2006); Robust Portfolio Management and Optimization (Wiley, 2007); and Quantitative Equity Investing: Techniques and Strategies (Wiley, 2010).
Petter is a frequent speaker, panelist and moderator at academic and industry conferences and events. He is a member of the editorial boards of the International Journal of Portfolio Analysis and Management (IJPAM), Journal of Financial Data Science (JFDS), Journal of Investment Strategies (JoIS), and Journal of Portfolio Management (JPM). Petter is an Advisory Board Member of Alternative Data Group (ADG), AISignals and Operations in Trading (Aisot), Betterment (one of the largest robo-advisors) and Volatility and Risk Institute at NYU Stern. He is also on the Board of Directors of the International Association for Quantitative Finance (IAQF) and Scientific Advisory Board Member of the Artificial Intelligence Finance Institute (AIFI).
As an advisory board member, consultant, and expert witness, Petter has provided services in areas including alternative data, data science, econometrics, forecasting models, high frequency trading, machine learning, portfolio optimization with transaction costs, quantitative and systematic trading, risk management, robo-advisory, smart beta strategies, trading strategies, transaction costs, and tax-aware investing.
He holds a Ph.D. in Mathematics from Yale University; an M.Phil. in Applied Mathematics from the Royal Institute of Technology, Stockholm, Sweden; and an M.S. in Mathematics from ETH Zurich, Switzerland

Terry Benzschawel:
Founder and Principal, Benzschawel Scientific, LLC
Terry Benzschawel: Founder and Principal, Benzschawel Scientific, LLC
Terry Benzschawel is the Founder and Principal of Benzschawel Scientific, LLC. The former Managing Director in Citigroup’s Institutional Clients Business. Terry headed the Credit Trading Analysis group which develops and implements quantitative tools and strategies for credit market trading and risk management, both for Citi’s clients and for in-house applications. Some sample tools include models of corporate default and recovery values, relative value of corporate bonds, loans, and credit default swaps, credit portfolio optimization, credit derivative trades, capital structure arbitrage, measuring and hedging liquidity risk, and cross-credit-sector asset allocation.
After six years of post-doctoral research in academia and industry and two years in consumer banking, Terry began his investment banking career in at Salomon Brothers in 1992. Terry built models for proprietary arbitrage trading in bonds, currencies and derivative securities in Salomon’s Fixed Income Arbitrage Group. In 1998, he moved to the Fixed Income Strategy department as a credit strategist with a focus on client-oriented solutions across all credit markets and has worked in related roles since then. Terry was promoted to Managing Director at Citi in 2008.
Terry received his Ph.D. in Experimental Psychology from Indiana University (1980) and his B.A. (with Distinction) from the University of Wisconsin (1975). Terry has done post-doctoral fellowships in Optometry at the University of California at Berkeley and in Ophthalmology at the Johns Hopkins University School of Medicine and was a visiting scientist at the IBM Thomas J. Watson Research Center prior to embarking on a career in finance. He currently serves on the steering committees of the Masters of Financial Engineering Programs at the University of California at Berkeley and the University of California at Los Angeles and Carnegie Mellon University’s Computational Finance Program.
Terry is a frequent speaker at industry conferences and events and has lectured on credit modelling at major universities. In addition, he has published over a dozen articles in refereed journals and is author of CREDIT MODELING: FACTS, THEORIES AND APPLICATIONS. In addition, Terry has been the instructor for courses in credit modelling for Incisive Media and the Centre for Finance Professionals. Finally, Terry has taught a course on credit modelling at Russia’s Sberbank in Moscow.
- Defining model risk and model uncertainty
- Overview of relevant regulatory frameworks
- Measuring uncertainty with ML
- Model risk of AI
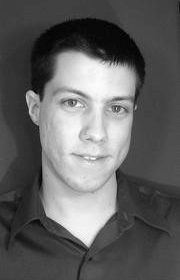
Victor Davis:
Python Developer, Yields.io
Victor Davis: Python Developer, Yields.io
Victor develops applications for machine learning and data analytics platforms. His background is in math, software development and data science. He currently works as a python developer for the FinTech startup Yields.io building tools for model development and validation.
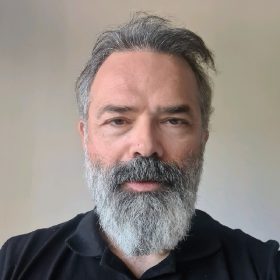
Miquel Noguer Alonso:
Co – Founder and Chief Science Officer, Artificial Intelligence Finance Institute – AIFI
Miquel Noguer Alonso: Co – Founder and Chief Science Officer, Artificial Intelligence Finance Institute – AIFI
Miquel Noguer is a financial markets practitioner with more than 20 years of experience in asset management, he is currently Head of Development at Global AI ( Big Data Artificial Intelligence in Finance company ) and Head on Innovation and Technology at IEF.
He worked for UBS AG (Switzerland) as Executive Director.for the last 10 years. He worked as a Chief Investment Office and CIO for Andbank from 2000 to 2006.
He is professor of Big Data in Finace at ESADE and Adjunct Professor at Columbia University teaching Asset Allocation, Big Data in Finance and Fintech. He received an MBA and a Degree in business administration and economics in ESADE in 1993. In 2010 he earned a PhD in quantitative finance with a Summa Cum Laude distinction (UNED – Madrid Spain).
We describe a framework for processing market data to produce short-term price forecasts for optimizing trade execution in futures and interest rate markets. The framework first computes a substantial number of simple features based on order book data. It then determines a number of signals from the features, using a variety of techniques including machine learning. Finally, a consensus layer uses a new clustering algorithm to decide which combination of signals to believe in which market conditions. The framework is in production use and is delivering substantial value.
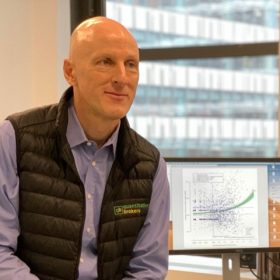
Robert Almgren:
Co-Founder and Head of Research, Quantitative Brokers
Robert Almgren: Co-Founder and Head of Research, Quantitative Brokers
Robert Almgren, co-founder and Head of Research at Quantitative Brokers, providing agency algorithmic trade execution and transaction cost measurement in US Treasury notes and bonds, as well as a broad range of futures products. Until 2008, Dr Almgren was a Managing Director and Head of Quantitative Strategies in the Electronic Trading Services group of Bank of America. Before that, he was a professor of mathematics at the University of Chicago and the University of Toronto, directing graduate programs in financial mathematics at both. Dr. Almgren holds a Ph.D. in Applied and Computational Mathematics from Princeton University. He has an extensive research record in applied mathematics, including papers on optimal trading, transaction cost measurement, and portfolio construction. He is a visiting professor at Princeton University, where he teaches a course on High Frequency Trading.
Quantitative Brokers http://www.quantitativebrokers.com
Topics:
- What is the current state of utilisation of machine learning in finance?
- What are the distinct features of machine learning problems in finance compared to other industries?
- What are the best practices to overcome these difficulties?
- What’s the evolution of a team using machine learning in terms of day to day operations?
What is a typical front office ‘Quant’ skillset going to look like in three to five years time? - How do we deal with model risk in machine learning case?
- How is machine learning expected to be regulated?
What applications can you list among its successes?
How much value is it adding over and above the “classical” techniques such as linear regression, convex optimisation, etc.? - Do you see high-performance computing (HPC) as a major enabler of machine learning?
What advances in HPC have caused the most progress? - What do you see as the most important machine learning techniques for the future?
What are the main pitfalls of using Machine Learning currently in trading strategies? - What new insights can Machine Learning offer into the analysis of financial time series?
Discuss the potential of Deep Learning in algorithmic trading? - Do you think machine learning and HPC will transform finance 5-10 years from now?
If so, how do you envisage this transformation? - Can you anticipate any pitfalls that we should watch out for.
- Discuss quantum computing in quant finance:
- Breakthroughs
- Applications
- Future uses
Panellists:

Terry Benzschawel:
Founder and Principal, Benzschawel Scientific, LLC
Terry Benzschawel: Founder and Principal, Benzschawel Scientific, LLC
Terry Benzschawel is the Founder and Principal of Benzschawel Scientific, LLC. The former Managing Director in Citigroup’s Institutional Clients Business. Terry headed the Credit Trading Analysis group which develops and implements quantitative tools and strategies for credit market trading and risk management, both for Citi’s clients and for in-house applications. Some sample tools include models of corporate default and recovery values, relative value of corporate bonds, loans, and credit default swaps, credit portfolio optimization, credit derivative trades, capital structure arbitrage, measuring and hedging liquidity risk, and cross-credit-sector asset allocation.
After six years of post-doctoral research in academia and industry and two years in consumer banking, Terry began his investment banking career in at Salomon Brothers in 1992. Terry built models for proprietary arbitrage trading in bonds, currencies and derivative securities in Salomon’s Fixed Income Arbitrage Group. In 1998, he moved to the Fixed Income Strategy department as a credit strategist with a focus on client-oriented solutions across all credit markets and has worked in related roles since then. Terry was promoted to Managing Director at Citi in 2008.
Terry received his Ph.D. in Experimental Psychology from Indiana University (1980) and his B.A. (with Distinction) from the University of Wisconsin (1975). Terry has done post-doctoral fellowships in Optometry at the University of California at Berkeley and in Ophthalmology at the Johns Hopkins University School of Medicine and was a visiting scientist at the IBM Thomas J. Watson Research Center prior to embarking on a career in finance. He currently serves on the steering committees of the Masters of Financial Engineering Programs at the University of California at Berkeley and the University of California at Los Angeles and Carnegie Mellon University’s Computational Finance Program.
Terry is a frequent speaker at industry conferences and events and has lectured on credit modelling at major universities. In addition, he has published over a dozen articles in refereed journals and is author of CREDIT MODELING: FACTS, THEORIES AND APPLICATIONS. In addition, Terry has been the instructor for courses in credit modelling for Incisive Media and the Centre for Finance Professionals. Finally, Terry has taught a course on credit modelling at Russia’s Sberbank in Moscow.
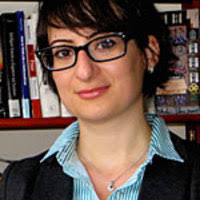
Knarig Arabshian:
Senior Knowledge Engineer in Technology Innovation, Federal Reserve Bank of New York
Knarig Arabshian: Senior Associate Knowledge Engineer in Technology Innovation, Federal Reserve Bank of New York
I am a Senior Associate Knowledge Engineer in Technology Strategy & Innovation at theFederal Reserve Bank of New York where I conduct research in semantic web technologies and text analytics for structuring financial data.
Previously, I was an Assistant Professor in the Computer Science Department at Hofstra University in Hempstead, NY and a Member of Technical Staff at Bell Labs in Murray Hill, NJ. I have also taught as an Adjunct Professor at Columbia University twice. I received my PhD in Computer Science from Columbia University in 2008, where I worked in theIRT Lab under the advisment of Henning Schulzrinne.
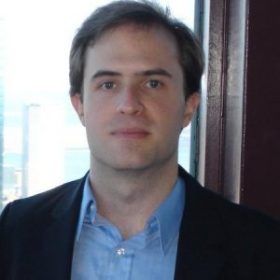
Gordon Ritter:
Founder, CIO, Ritter Alpha, LP
Gordon Ritter: Founder, CIO, Ritter Alpha, LP
Gordon Ritter completed his PhD in mathematical physics at Harvard University in 2007, where his published work ranged across the fields of quantum computation, quantum field theory, differential geometry and abstract algebra.
Prior to Harvard he earned his Bachelor’s degree with honours in Mathematics from the University of Chicago. Gordon is currently a senior portfolio manager at GSA Capital, and leader of a team trading a range of high-Sharpe absolute return strategies across geographies and asset classes. GSA Capital has won the Equity Market Neutral & Quantitative Strategies category at the Eurohedge awards four times, with numerous other awards including in the long-term performance category.
Prior to joining GSA, Gordon was a Vice President of Highbridge Capital and a core member of the firm’s statistical arbitrage group, which although less than 20 people, was one of the most successful quantitative trading groups in history, responsible for billions in pro_t and trillions of dollars of trades across equities, futures and options.
Concurrently with his positions in industry, Gordon teaches courses ranging from portfolio management to econometrics, continuous-time finance, and market microstructure in the Department of Statistics at Rutgers University, and also in the MFE programs at Baruch College (CUNY) and New York University (both ranked in the top 5 MFE programs).
He has published several articles on modern portfolio theory in top practitioner journals including Risk, and academic journals including European Journal of Operational Research.
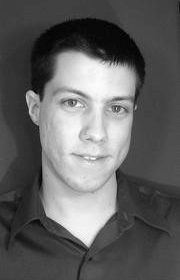
Victor Davis:
Python Developer, Yields.io
Victor Davis: Python Developer, Yields.io
Victor develops applications for machine learning and data analytics platforms. His background is in math, software development and data science. He currently works as a python developer for the FinTech startup Yields.io building tools for model development and validation.
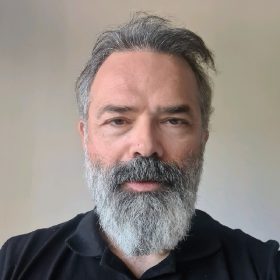
Miquel Noguer Alonso:
Co – Founder and Chief Science Officer, Artificial Intelligence Finance Institute – AIFI
Miquel Noguer Alonso: Co – Founder and Chief Science Officer, Artificial Intelligence Finance Institute – AIFI
Miquel Noguer is a financial markets practitioner with more than 20 years of experience in asset management, he is currently Head of Development at Global AI ( Big Data Artificial Intelligence in Finance company ) and Head on Innovation and Technology at IEF.
He worked for UBS AG (Switzerland) as Executive Director.for the last 10 years. He worked as a Chief Investment Office and CIO for Andbank from 2000 to 2006.
He is professor of Big Data in Finace at ESADE and Adjunct Professor at Columbia University teaching Asset Allocation, Big Data in Finance and Fintech. He received an MBA and a Degree in business administration and economics in ESADE in 1993. In 2010 he earned a PhD in quantitative finance with a Summa Cum Laude distinction (UNED – Madrid Spain).